#16: A fraction of great things you missed this year
This week: Death contours, the twin baby boom, good conversations, music and languages, blood pressure drugs, conspiracy theories, Tasmanian devil cancers, cheating viruses, and more.
This is my sixteenth post of Scientific Discovery, a newsletter where I’ll share great new scientific research that you may have missed. Check out the About page if you’re interested in why I’m writing this.
Hello! It’s been almost six months since I’ve done a round up of great research I’ve read, so this post is long & won’t fit on email – but it still only covers a fraction of the things I wanted to share. I hope you enjoy it! If you spot any errors, let me know so I can fix them.
Recently I’ve been thinking about charts that have helped me see something in a new light, and that illustrate an important concept. Here’s one of them.
The contours of death
Okay, I just enjoy giving spooky titles to my subheadings, but this chart’s very interesting.
It shows death rates in the US across two dimensions: time and age.
The shades represent death rates, and splodges show when death rates have risen. The contour lines around the splodges are like an elevation map: they show how much death rates have risen (or fallen).1 You can see how they’ve risen and fallen over time (on the x-axis) and across age groups (on the y-axis).
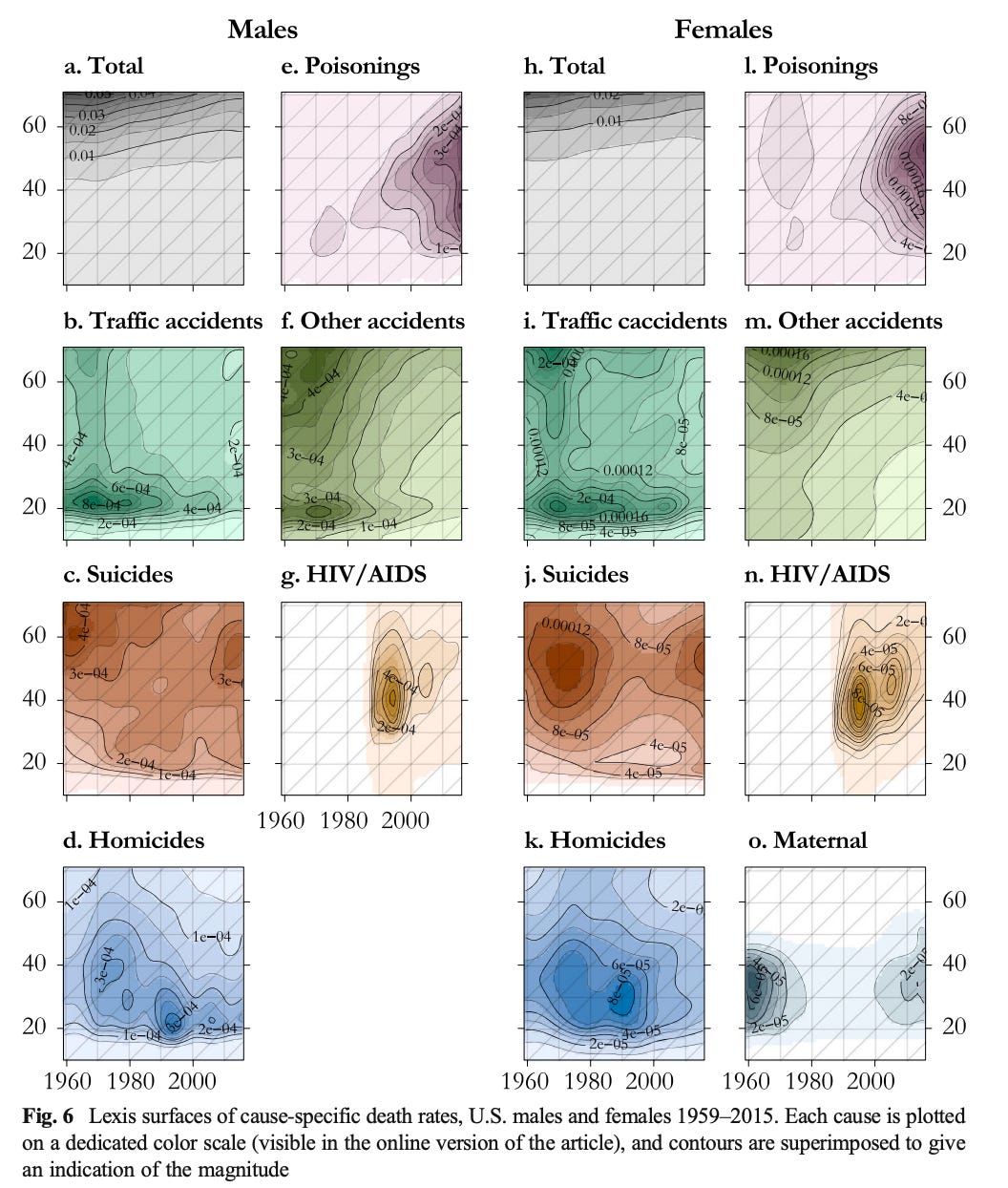
You’ll see that events with wider splodges occurred over longer time periods. Events with taller splodges affected many age groups.
For example, look at ‘poisonings’, which includes substance use and overdoses. Here, it shows the opioid crisis, and you can see it rising gradually across adult age groups.
Or look at the HIV/AIDS panel: as an exponentially-growing epidemic, it surged over a very short period of time, especially among young and middle-aged adults.
Or look at traffic accidents, which were especially deadly among young people and the elderly, but have become less so over time.
Suddenly, the plots I’m used to seeing – line charts where something rises for some age groups – seem quite flat, even when they show the same data.
The shapes show how events permeate across a population and time. I think they also give visual clues about the nature of the underlying events: Were they constrained to only affecting a particular age group? Did they grow exponentially over time? And maybe these tell us something about how and why they happened.
I really liked this chart, and could look at it for a long time. Are there other types of charts that have helped you understand some data better?
Here are some little dives into other great research I’ve read!
The twin baby boom
This new database, called the Human Multiple Births Database, shows national data on twin births from 24 countries. In the chart below you can see trends in having twins, measured as a rate per 1,000 deliveries.
Twin rates rose from the 1970s across many countries. You can see that they approximately doubled in some countries, from around 10 twins per 1,000 deliveries to 20 or more.
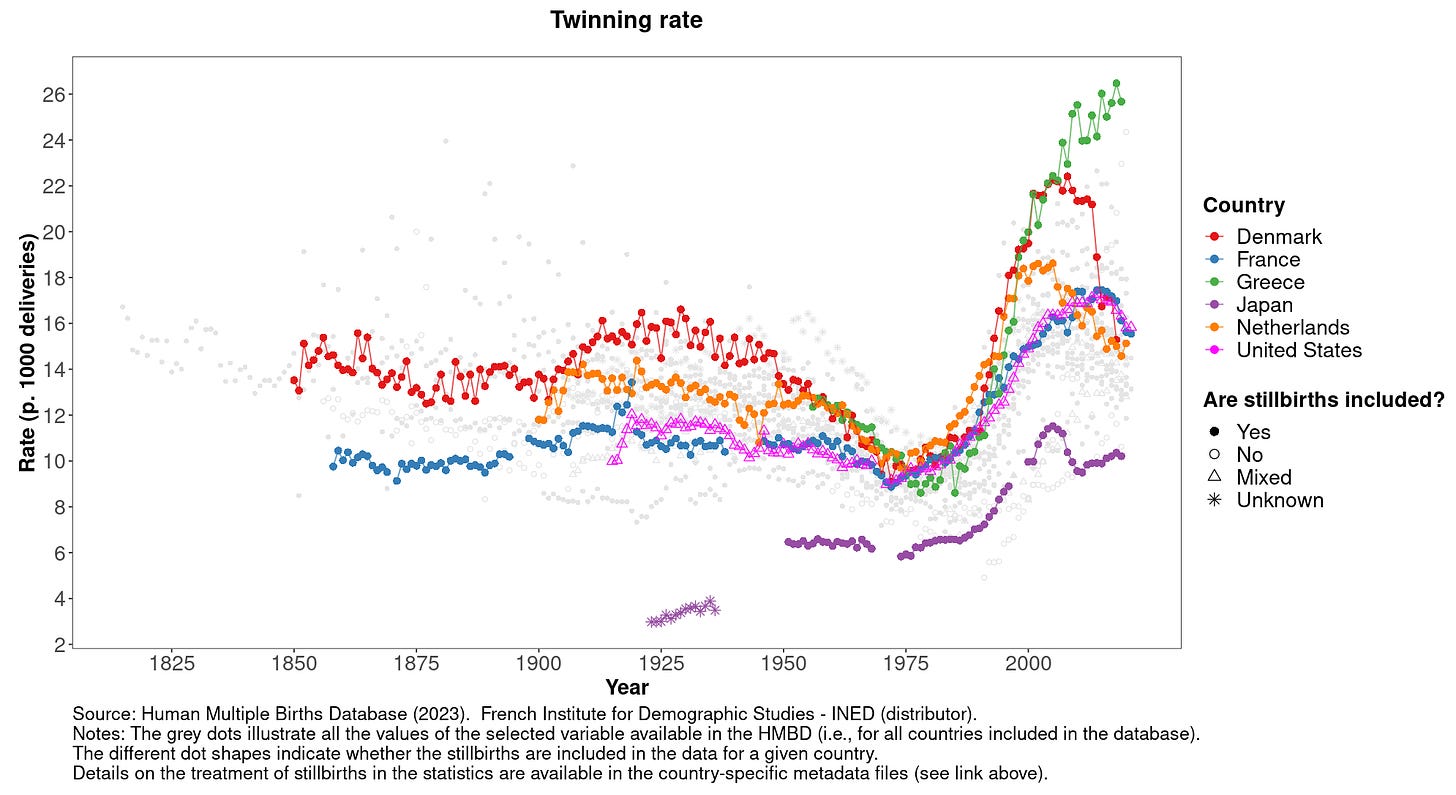
The main reason for the rise is because women have been having children later on average and using reproductive technologies that were invented then. This includes IVF (where multiple eggs are implanted) and ovarian stimulation (where multiple eggs are released) to make sure that at least one is successful. These can result in having fraternal twins.
But since the early 2000s, twin rates have plateaued and fallen. This has happened in a range of countries, as they introduced new standards for reproductive technologies. (Many now countries measure the success of these technologies by their effect on singleton2 live births, because pregnancies with multiple babies tend to be riskier.)
I’d learnt about this at school, but seeing the trend helped me understand the magnitude of twin trends in the population. You can also explore the data for other countries on this interactive website by the researchers.
What makes a good conversationalist?
Surprisingly, the pandemic provided an opportunity to study this question in detail.
Volunteers in this new study had unscripted freeform conversations with strangers over Zoom in 2020, in the United States. They were asked to ‘talk about whatever you like, just imagine you have met someone at a social event and you’re getting to know each other.’
The conversations were video recorded, and analyzed for patterns of speech and behavior. Then, their speech patterns were compared to people’s feelings before and after the conversation, and their ratings of their conversational partner. In total, the dataset had 1,656 conversations each lasting at least 25 minutes.
Since the dataset was large and detailed, it could answer a lot of questions. First, how long are the gaps between each person’s speech in a conversation? Answer: Less than a second on average, which suggests that people predict when their conversational partner’s sentences are going to end and how they’ll respond. Some people spoke too soon and their speech slightly overlapped or they interrupted the other person.
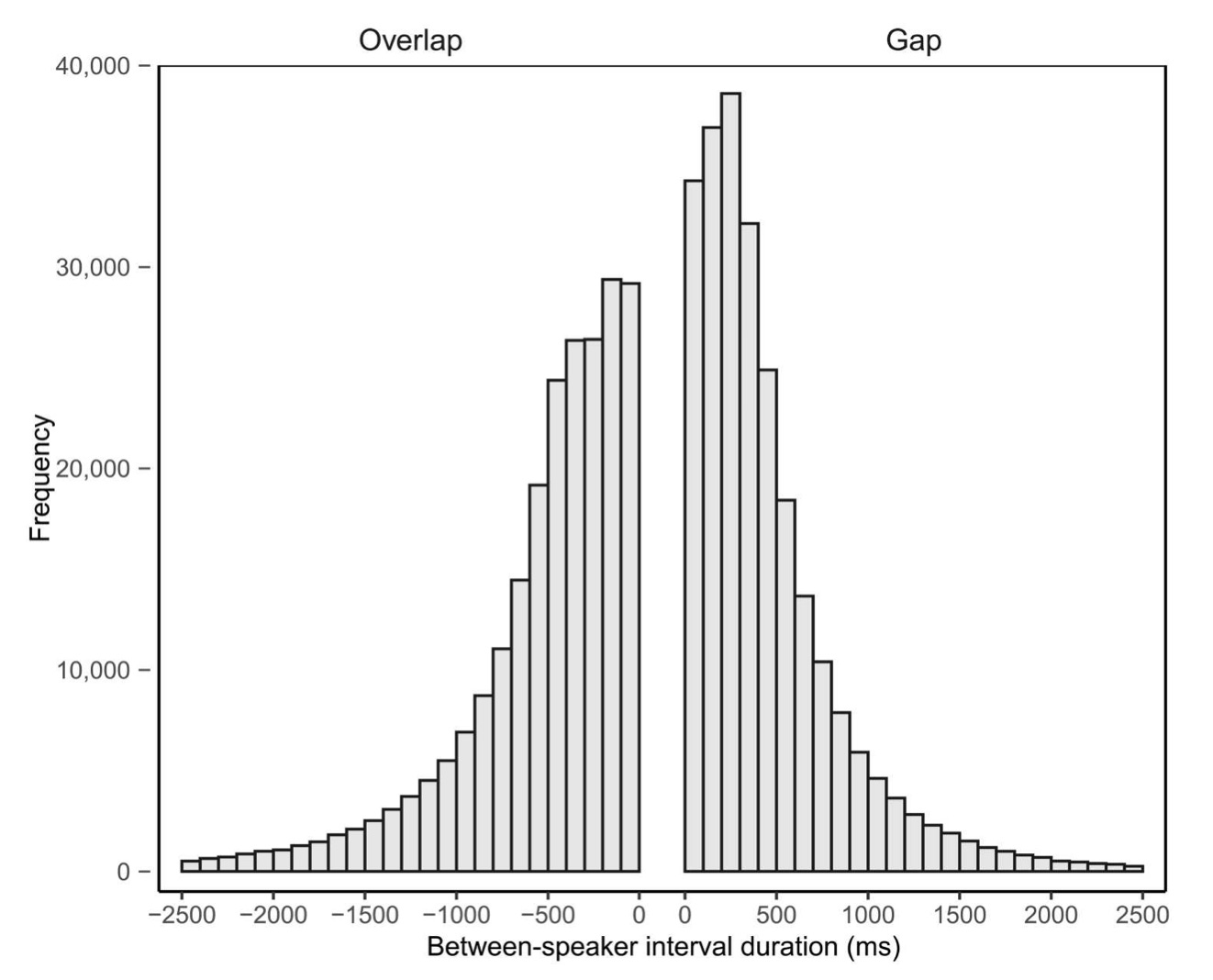
What are the most common ‘backchannel words’? (These are words people use to show they’re following the other person’s speech.) Answer: Yeah and mhm. This study was done in the US, and it’s likely these vary in other countries.
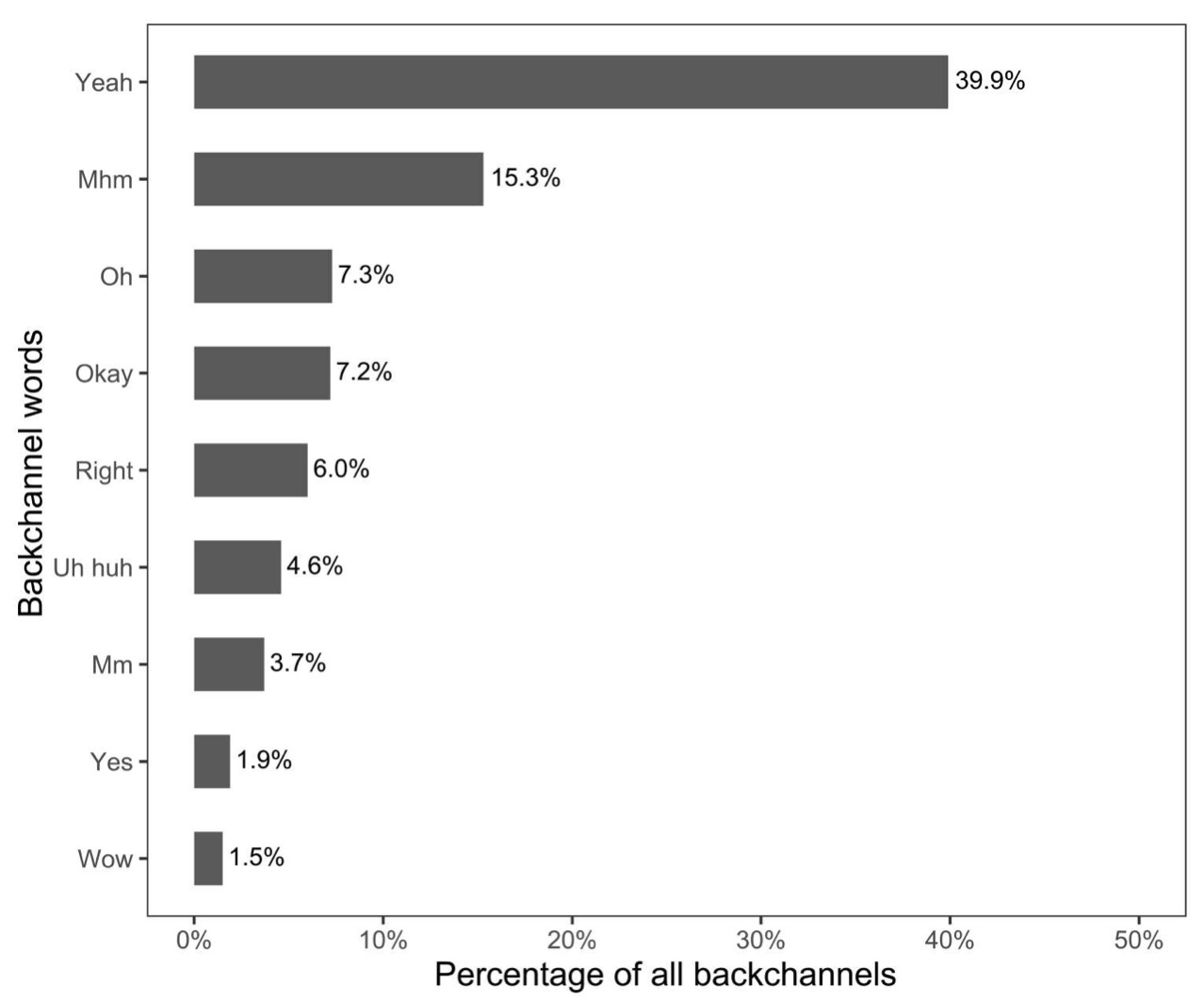
Were people happier after a freeform conversation with a stranger? Yes, on average! You can see this across age groups below. Volunteers were asked to rate their happiness on a 9-point scale immediately before and after the conversation.
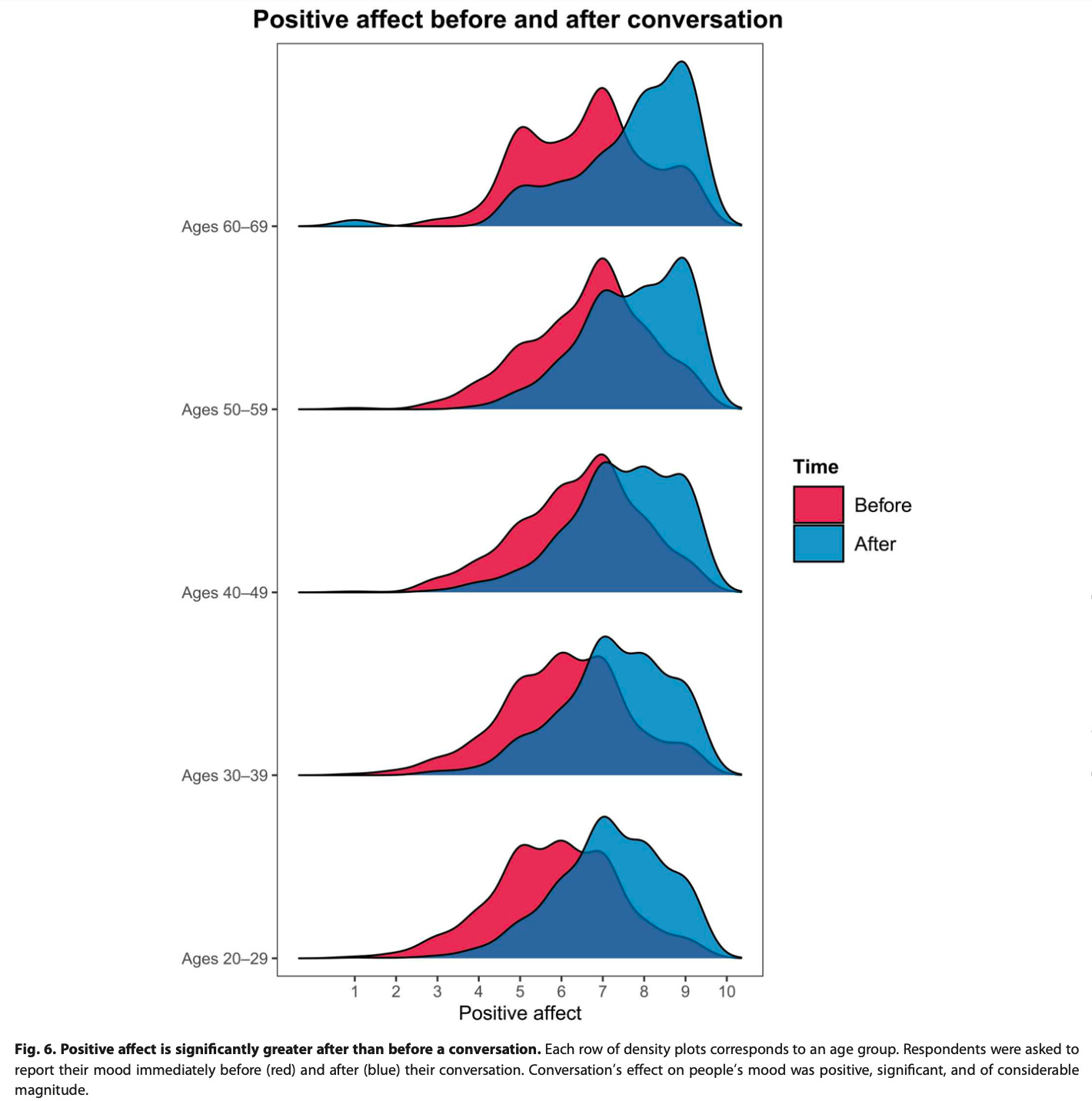
Although I wonder how much this came from the sample – people who like having conversations were probably more likely to join the study, and it happened during the pandemic, when many people were probably very bored at home.
Finally, what makes a good conversationalist?
Volunteers were asked: ‘Imagine you were to rank the last 100 people you had a conversation with according to how good of a conversationalist they are. ‘0’ is the least good conversationalist you’ve talked to. ‘50’ is right in the middle. ‘100’ is the best conversationalist. Where would you rank the person that you just talked to on this scale?’
People who were rated higher by their conversational partners tended to speak fairly quickly, and with more emotional intensity. Also, they tended to use more head movement (nodding for yes and shaking for no) while listening, and showed more facial signs of happiness.3
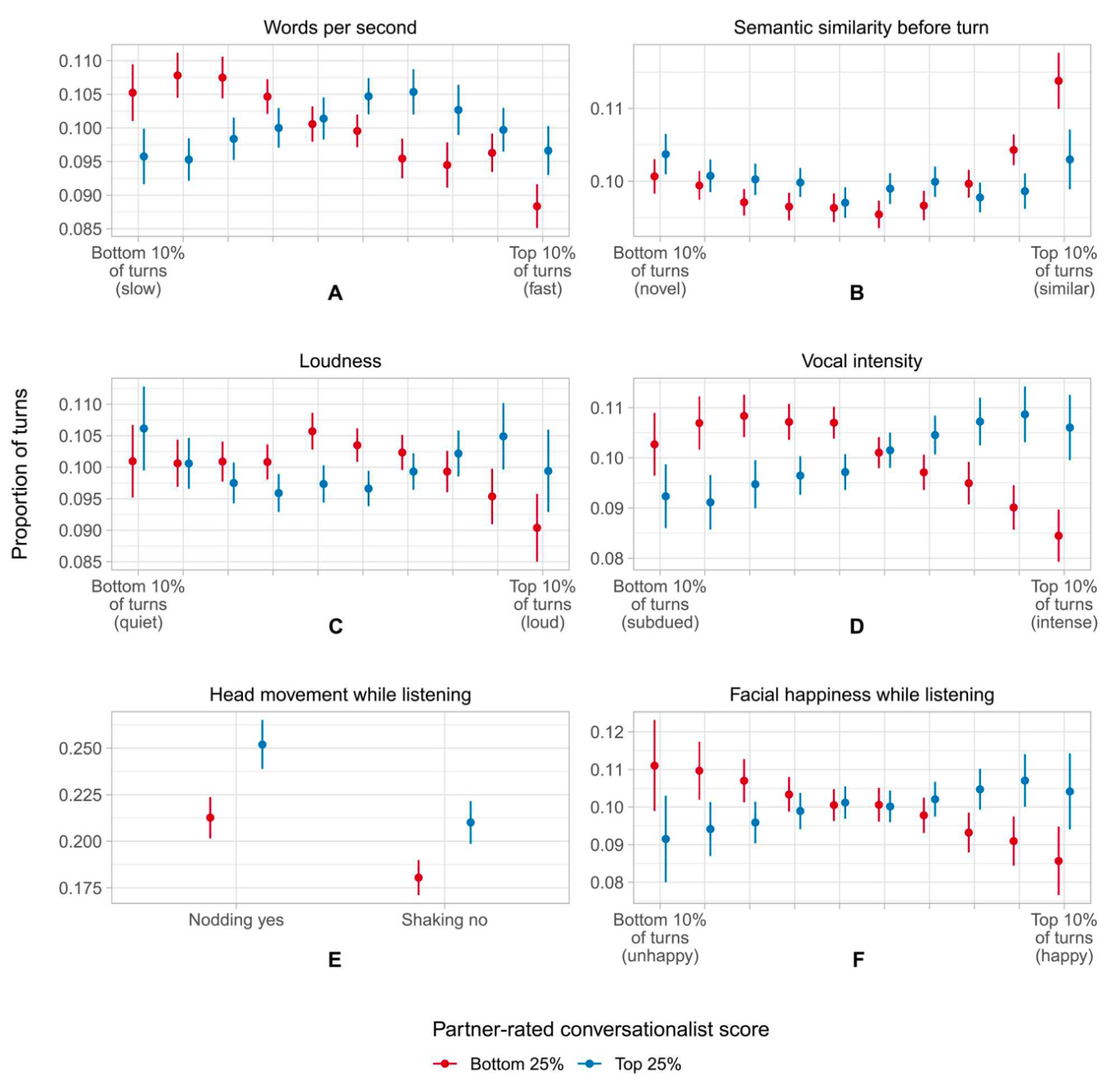
I really liked this paper because it described many basic aspects of conversations, and compared the analysis across different pattern-recognition software.
Here’s something else cool.
Are speakers of tonal languages better at discerning music?
You might know that I grew up in Hong Kong, where most people speak Cantonese.
It’s considered a difficult language to learn to speak because it’s a ‘tonal language’ – each syllable can be spoken in 6 different tones.4 This means the pitch of a syllable can be either rising, falling, stable, or falling-then-rising; and can start at different levels.
The tone provides information about the word: if you say a word with the wrong tone, people might think you’ve said a completely different word. Here’s an example:
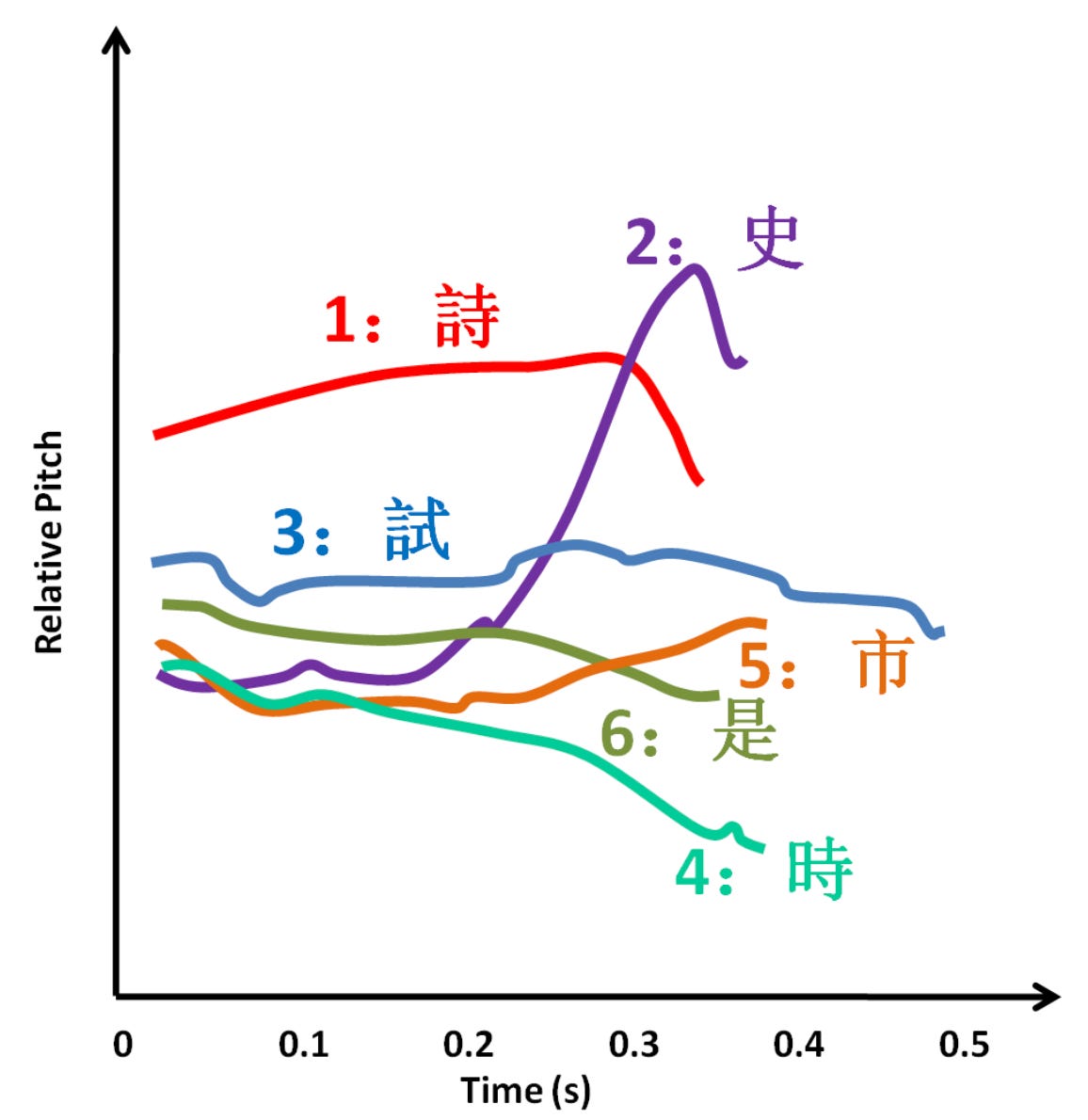
I listen to Cantopop and Vietpop music sometimes5, and I’ve occasionally wondered about the relationship between music and tones: Do tones constrain the compositions that songwriters can make? Are tonal speakers better at matching a pitch?
A new study looks at this topic. The researchers created a music game (here’s the link) to test if people could notice slight changes in melody, tuning, and beats. It was played by 493,100 participants around the world, who spoke 54 different languages in total.
They found that people who spoke tonal languages – like Mandarin, Cantonese and Vietnamese – generally tended to do better at distinguishing melodies, but weren’t as good as others at perceiving beats. You can see this below.
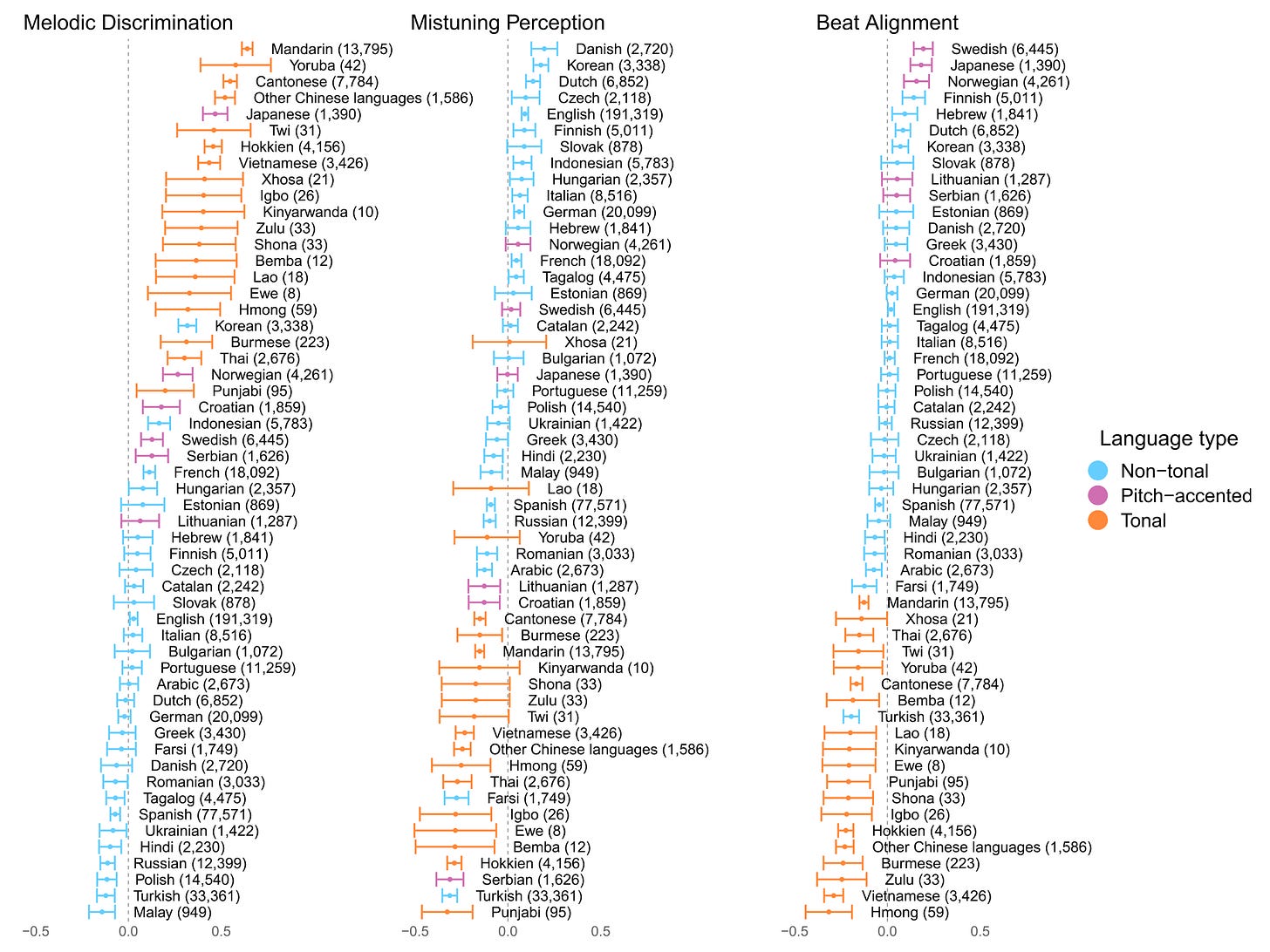
The authors suggest maybe this is because they place more weight on cues that are relevant to their language.
When I read studies like this, I try to imagine the different kinds of people who might be participants – could the participants vary on skills relevant to the game? Could their skills vary between countries or languages, for other reasons?
Here the study was an online game. So I’m thinking: maybe people from some cultures are more likely to play musical instruments, or the game got shared on music forums in some countries. Also, maybe people speaking some languages understood the game instructions better, or were more familiar with the melodies or rhythms in the game.
This study adjusted and explored several of these factors. You can see below that people who have taken music lessons performed better on all three parts of the game, across language types.
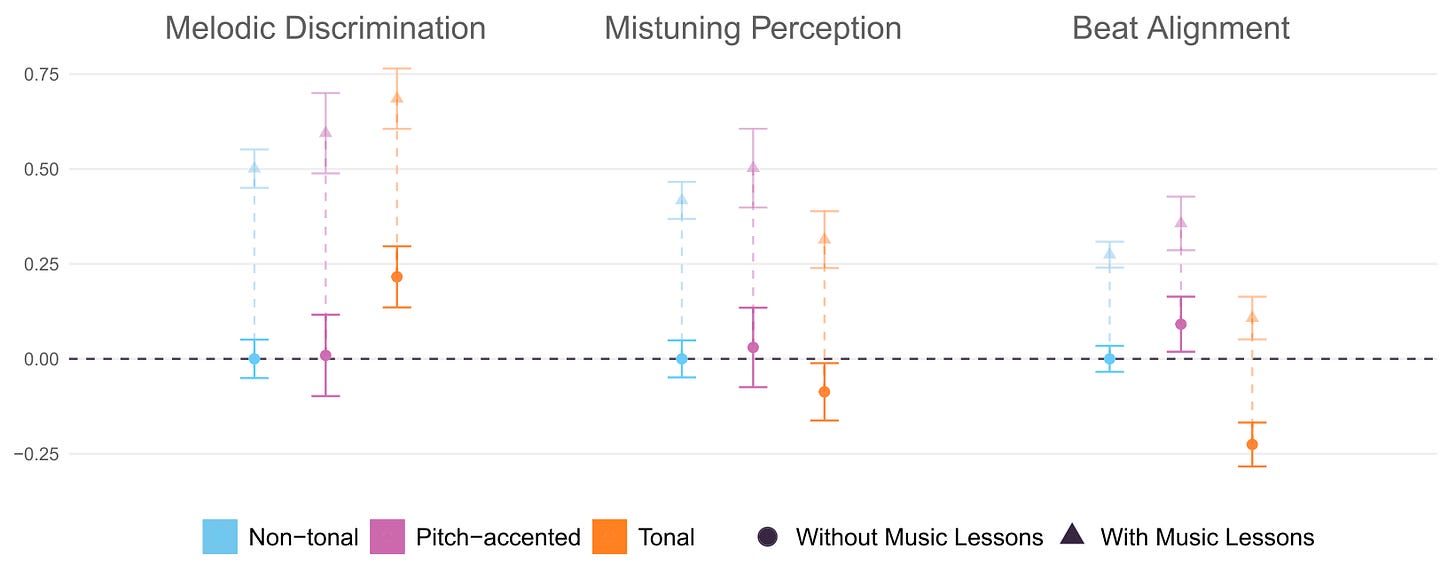
The researchers say that “in each case, the effect size associated with being a tonal language speaker was roughly half as large as the effect of receiving music lessons, indicating an effect of substantive practical significance.”
I think that’s a good way to put the advantage into context! It’s also cool to use a large online game in research, to get enough people from different backgrounds, and where you can fine-tune parts of the experiment.
Do blood pressure drugs’ effects vary between people?
Lots of research is focused on understanding the average effects of treatments.
In a randomized controlled trial, each person is allocated to only one of the groups at a time. So we don’t get to see whether they, specifically, would have gotten better anyway. We only get to see the change for people on average.
When we try to understand whether something works, it can be challenging to tell if it works for everyone to the same degree or if it works more for some people and less for others.
The main reason for this is that we’re only able to see one version of history: When someone makes a decision, we can see what follows, but we can’t see what would’ve happened if they had made a different decision.
This distinction – between averages and individuals – is blurrier when people naturally experience a lot of fluctuations. For example, there’s a lot of day-to-day variation in people’s blood pressure – so maybe for some people who’ve taken a medication, it was just a good day, and for others it was a bad day.
But there are ways to get understand how much the effect of a drug varies between people.
One way is by understanding the mechanism of the drug. In cancer treatment, for example, many cancer patients receive treatment for their specific cancer mutations, after genetic testing on their tumours.
Another way is by testing the effect in different subgroups, although you might need very large sample sizes to find the precise differences in the drug’s effect in the different subgroups.
A third way is to track people’s fluctuations while theyʼre using the same drug, and adjust for this fluctuation. This helps to see the probability that people would do better anyway, without the drug.
That’s what this new RCT did. 280 patients with hypertension were randomly allocated to each of four different drugs (one after the other), and then two of the same drugs again. Their blood pressure was measured 14 times a day. The drugs were lisinopril, candesartan, hydrochlorothiazide and amlodipine; each drug is used to reduce blood pressure, but comes from a different drug class.
Let’s see the results! (This chart looks complicated but I’ll explain it below.)
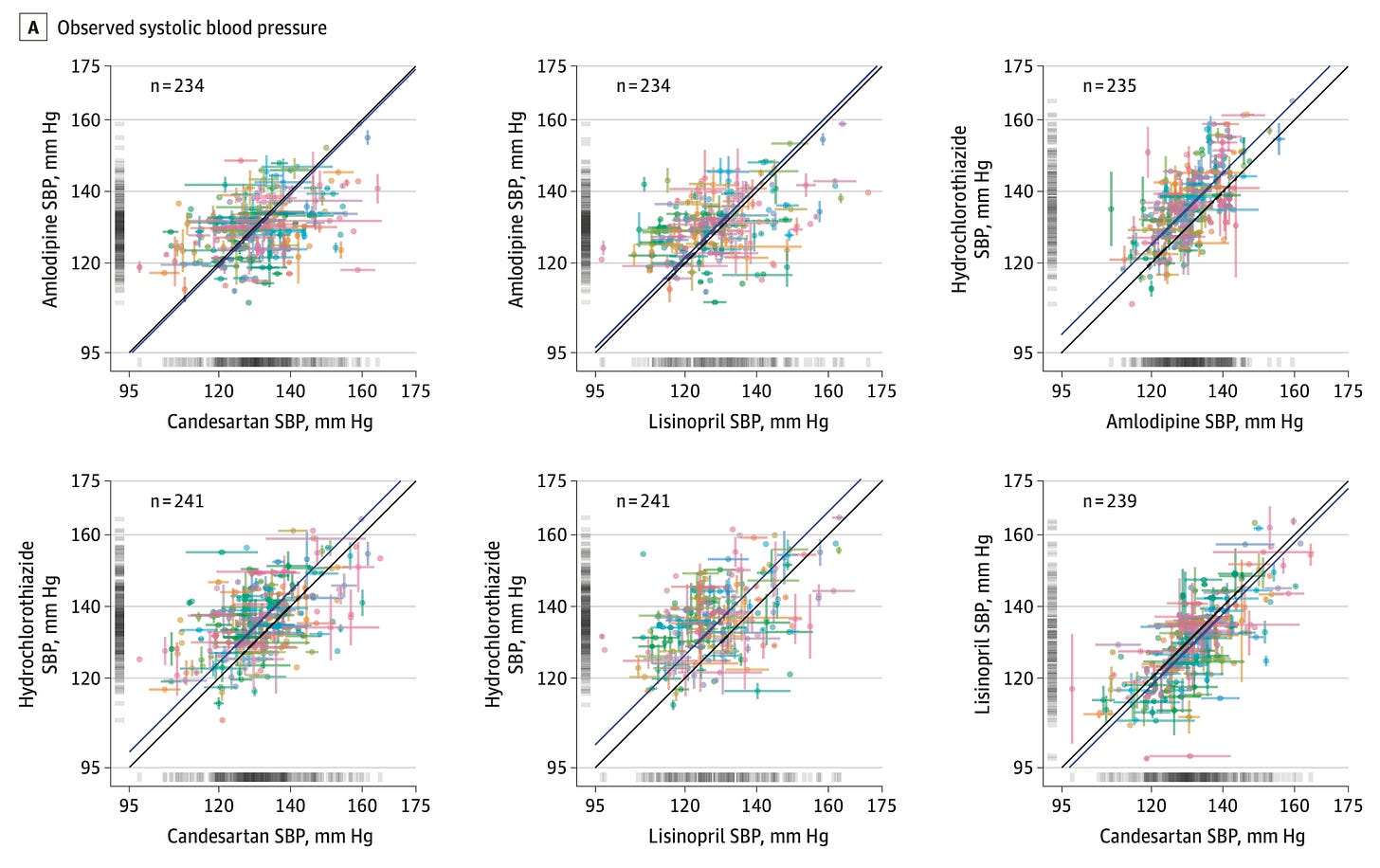
Each panel compares two drugs to each other. The blue diagonal line compares people’s blood pressure on one drug to their blood pressure on another drug. The black diagonal line shows where it would be if there was no difference between the drugs.
You can see that for some pairs of drugs, the two lines are more apart, and there are differences in drug efficacy. For example, patients taking hydrochlorothiazide have a 5.1 mmHg higher blood pressure than those taking amlodipine, on average. Lisinopril was the most effective, on average.
Each dot represents a participant. Some dots have a range – this is because the participant was given the same drug twice, and the range shows the variation in their blood pressure over the repeated drug. You can see that there’s often a lot of variation within the same people.
Because of the design of the trial, the researchers could also test whether different people did better on some drugs than others. With this, they estimated that if the participants received personalized treatment with a single drug that worked better for them, they’d have a 4.4 mmHg lower blood pressure on average. They also estimated that personalized treatment would be better than if all of them received the most effective drug (lisinopril).
Even more
Here’s a more condensed summary of some other great stuff I’ve read.
Can we save Tasmanian devils from contagious cancers?
Something you may not have heard of. The population of Tasmanian devils has dropped by around two-thirds in the last 25 years. They’ve been affected by facial cancers, which spread when they bite each other during fights.
The declining population and density of Tasmanian devils, and the rise of the Devil Facial Tumour (DFT1 & 2). The spread of the cancers may now be slowing down because of lower population density of the devils, and some adaptation, but they’re still vulnerable. Source: Quantifying 25 years of disease-caused declines in Tasmanian devil populations: host density drives spatial pathogen spread (Calum X. Cunningham et al., 2021) There are two types of these cancers, called Devil Facial Tumour 1 and 2. They each cause very similar disease, but a recent study shows that each type evolved independently. (This BBC article has more background and photos in case you’re interested.)
Now, field trials are about to begin to test vaccines that could protect them from these cancers.
The vaccines will be rolled out as oral bait, similar to how oral rabies vaccines have been given to wildlife across Europe (which happened by dropping them from helicopters and aircraft). I hope the devil cancer vaccines succeed!
A new study shows how estimates of people’s beliefs in conspiracy theories are often overestimated (Seth J. Hill and Margaret E. Roberts, 2023).
Estimates are usually based on surveys where people are asked whether they agree or disagree with a conspiratorial belief. Unfortunately people tend to prefer to agree across surveys, which is called ‘acquiescence bias’. This leads to overestimating how many people actually believe something.
The researchers show this bias exists by asking the same questions in multiple different styles, like randomly flipping the phrasing of the question so the agree/disagree responses would be flipped.
I also liked this quote from the study: “The consequences of bias increase in the rarity of the target belief. The smaller the fraction of the population that holds a belief—e.g., when asking about outlandish conspiracies—the more likely agreeable responses are due to acquiescence bias rather than actual belief.”
You might have heard of ‘left-digit bias.’ This is the idea that customers tend to treat prices like $3.99 as being much lower than $4.00.
Here’s an example from a recent paper (Strulov-Shlain, 2022). The author looks at how the demand for products changes as the price rises, by using data on price changes and sales of the same products over time, from around 3,600 stores in the US.
You can see this below. There are sudden drops in demand as prices reach a new dollar. The author estimates that customers treat a 1 cent rise at the boundary (from $3.99 to $4.00, for example) as a 20 cent rise.
Left-digit bias in customers’ perception of prices. Source: More than a penny’s worth: Left-digit bias and firm pricing (Avner Strulov-Shlain, 2023)
Did the 1918 flu pandemic cause a baby boom two years later, in 1920 Europe?
According to a 2004 study using data from Norway, the end of the 1918 Spanish flu pandemic led to a baby boom.
A new study challenges this view, and argues that it was actually the end of WWI (or rather the post-WWI economy) that led to the baby boom.
The authors look at more neutral countries, not just Norway, and show that communities with the biggest baby busts in 1919 also had baby busts in 1920. This is evidence against a rebound.
Communities that had lower birth rates in 1919 (compared to 1916–1918) also had lower birth rates in 1920. Source: Did the 1918 influenza pandemic cause a 1920 baby boom? Demographic evidence from neutral Europe. (Hampton Gaddy and Mathias Mølbak Ingholt) Another important point is that, while the 1918 flu pandemic was a global phenomenon, the 1920 baby boom was largely concentrated in Europe.
The crude birth rate (CBR) in countries in 1920, relative to 1915–17. Source: Did the 1918 influenza pandemic cause a 1920 baby boom? Demographic evidence from neutral Europe. (Hampton Gaddy and Mathias Mølbak Ingholt)
Have you heard of ‘multipartite viruses’? These are viruses that are made up of different segments that spread separately. But, when they infect new cells, they can only replicate their entire genome if all the different segments have infected the same cell.
This seems like a huge disadvantage – since most of the time, there won’t be all the segments in the same cell, so most lineages die out. So why have multipartite viruses evolved several times independently? Why don’t they all eventually die out?
A new study uses theoretical simulations to argue that they’ve evolved by cheating, and that there are more advantages to this than previously thought.
Here’s a simple summary as I understand it. First, there were regular ‘monopartite’ viruses without segments. If broken up segments infect the same cells, they can replicate along with the monopartite virus. Cheats have advantages: for example, they can replicate faster because they’re shorter. If different cheats (encoding different segments of the same virus) infect the same cells, they could eventually replace the whole population. Source: Cheating leads to the evolution of multipartite viruses (Asher Leeks, Penny Grace Young, Paul Eugene Turner, Geoff Wild, Stuart Andrew West, 2023)
What I’ve been upto
At Works in Progress, we recently launched a new platform for updateable books – specifically, Stewart Brand’s in-progress book ‘Maintenance: Of Everything’.
You might have read his fantastic piece The Maintenance Race last year. That was only the first chapter: here you can read the rest.
This platform lets you make comments and suggestions on the chapters, which he can incorporate into new versions later on. My colleague and friend Nick Whitaker did an amazing job making this happen.
At Our World in Data, I’ve been writing about the key things you need to know about big topics in global health, like influenza, diarrheal diseases, data on mental health, and suicides.
For example, did you know that there are multiple flu seasons each year in several tropical countries near the equator, or that they can have flu present all-year-round?
The fraction of flu tests that return positive. In Singapore and Thailand, flu is present all year round. Source: FluNet by the World Health Organization, via Our World in Data. I also wrote about one of the most common causes of death in children globally, that most people don’t know about: diarrheal diseases.
It’s estimated that 500,000 children under five die from them in a year, and three-quarters of these deaths are caused by 10 pathogens.
Diarrheal death rates are 50x higher in poor countries than wealthy countries, and most deaths could be prevented with measures like better sanitation, rotavirus vaccination, and oral rehydration therapy.
Aside from work, I’ve been eating lots of gelato – so much so that today my phone automatically created a collage of gelato selfies I had taken. I like this picture because it looks like I’m saying ‘Cheers!’ with a gelato.
I also recently went to the Hunterian museum. It’s about the history of surgery and anatomy, and is my favourite museum in London. It recently reopened, after being shut for 6 years for renovation. It’s amazing, but also quite disturbing, and maybe not recommended for people who get queasy around body parts (which might be most people).
Anyway, that’s all for now! I hope you liked reading this and learnt something new. If you haven’t already, you should subscribe! I have lots more planned to write.
See you next time! :)
– Saloni
Thanks very much to my friend Nathaniel Bechhofer for reading a draft of this post and pointing out some clumsy phrasings.
In case you’re there too, I recently joined Threads (and Bluesky). I don’t know, maybe none of these will work out, but maybe they will, and I’m a little fed up with twitter.
27th January 2024: I improved phrasing in several parts of this post to make it easier to read & understand.
15th July 2023: I corrected this sentence, which originally said that the contours showed when death rates doubled or halved. That was only the case for some panels, and the others had different scales.
Singleton is a term that describes non-twins.
10th July 2023: I rephrased this sentence to avoid giving the impression that these were causal effects.
Traditionally, people say Cantonese has 9 tones, but linguists often say that some of them are equivalent and there are only 6. I don’t know enough to have a strong view, so let’s just say it’s a lot of tones.
If you’re feeling like something new, here are a couple of playlists I made on Spotify, since the last one I shared was popular.
Great stuff, thank you, have a great summer! 💚 🥃
Stay curious!